Data science, machine learning and Artificial Intelligence have evolved and come a long way since the 50s. The development of computing power and data infrastructure facilitated this growth. Deep learning and neural networks are integral parts of AI and ML technology.
Interestingly, we use deep learning in many of our daily activities unknowingly. Neural networks are crucial for deep learning. Here, you’ll find a detailed rundown of these two essential concepts of big data.
What is Deep Learning?
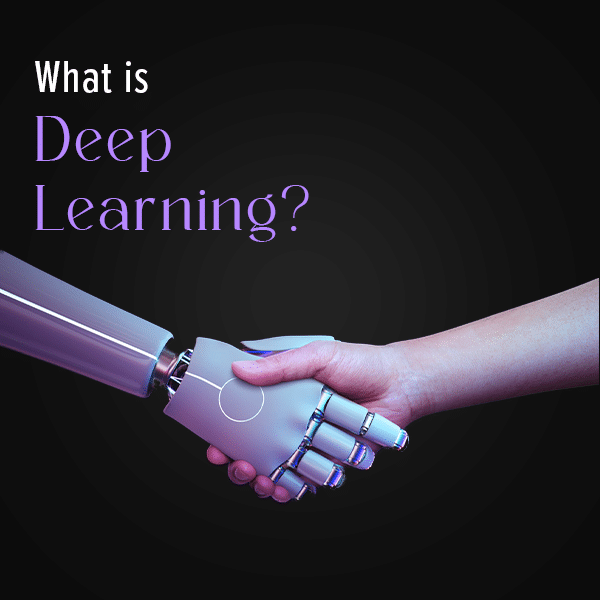
Deep learning refers to a machine learning training model that works very similarly to the human brain. The algorithms designed in the training model are not simple. Rather they are complex and ‘deep’ as they come with multiple layers that are used for processing.
Since deep learning functions like the human brain, it can handle a vast volume of data. Also, a large volume of data is necessary for training in this case. Notably, deep learning is capable of processing both unlabelled and unstructured data. It can also create more complicated statistical models, which tend to be more accurate.
What is a Neural Network?
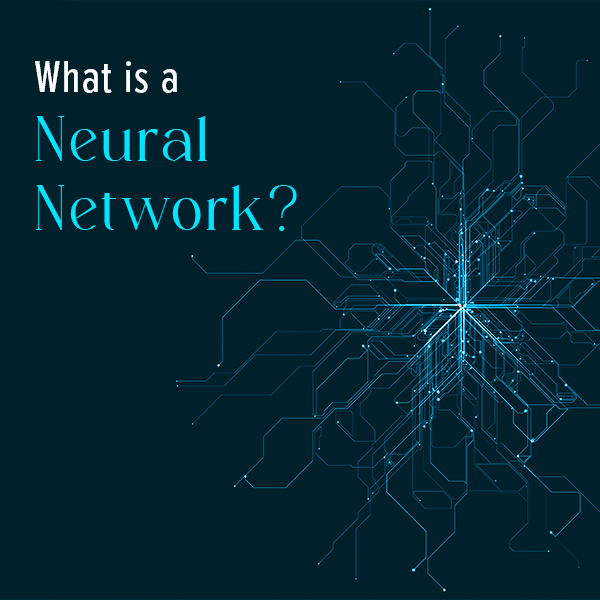
Artificial Neural Networks (ANN), or simply neural network, is a network that facilitates communication between the multiple layers in deep learning. It is a framework which consists of multiple machine learning algorithms.
This framework acts like a web of interconnected nodes similar to the neurons of the human brain. Neural networks can identify patterns and correlations in a data set to cluster and classify them. This helps them to learn and improve over time.
How Does Deep Learning Work?
Deep learning works in a manner that is the same as a human brain. It is provided with a vast volume of training data along with processing power. The learning model is made to develop the correct predictive models with vast unstructured and unlabelled data.
Let’s make this simple for you to comprehend. Suppose a child is learning how to identify a cat. The child will identify it by associating all those features that cats usually possess. The data engineers will provide training data for the model. The program will develop a predictive model with all provided information of a feature set for cats.
The model will analyse the pixel of an image and identify the tail, fur, whiskers, and ears and label it as a cat. However, with time and more data, the predictive model will become more accurate.
How Important are Neural Networks?
Neural networks play a crucial role in deep learning models. They help in relaying information from one layer to another, just as the neurons do in human brains. The neurons are the most fundamental unit of the neural network and the brain. They receive input, process it and pass it to the next neuron in the multiple hidden layers.
A basic neural network consists of three layers: input layer, hidden layer and output layer. These can be used to solve real-life problems and situations.
What are The Types of Deep Learning Networks?
The various types of deep learning networks include Recurrent Neural Networks, Convolutional Neural Networks, Feedforward Neural Networks, and Autoencoder Neural Networks.
Recurrent Neural Networks make use of a sequential framework. Convolutional Neural Networks make use of five layers. Feedforward has one perceptron of a layer connected to all perceptrons of the next layer. Auto encoders are used to develop encoders.
What are The Differences Between Deep Learning and Neural Networks?
While many often use deep learning and neural network interchangeably, they are two different concepts. These are two interconnected with one another but vary in terms of structure, time and accuracy, performance, applications and more.
-
Structure: Deep learning algorithms structurally consist of a motherboard, RAM, PSU and processors. Neural networks have neurons, propagation function, connection and weights.
-
Performance: Neural networks provide lower performance as compared to deep learning algorithms.
-
Time and Accuracy: It takes lesser time to train neural networks, while deep learning models take more time. Neural networks also have lower accuracy as compared to deep learning models.
-
Applications: Neural networks are used for pattern recognition, clustering, decision-making, machine learning, etc. Deep learning applications include speech recognition, natural language processing, social network filtering and more.
Wrapping up
Data science has evolved over the past few decades. With further improvements in technology, experts will take neural networks, deep learning and big data technology a notch up.