Data in its raw form is unstructured and practically useless for big businesses. Data visualisation is a useful technique for structuring and representing unstructured data. With DataViz, raw data can be communicated in a form that is understandable to humans. For any data science project development, the visualisation of data is a crucial task.
Interested to know the details of how data visualisation plays a vital part in data science projects? Let’s check what exactly it is.
What is Data Visualisation?
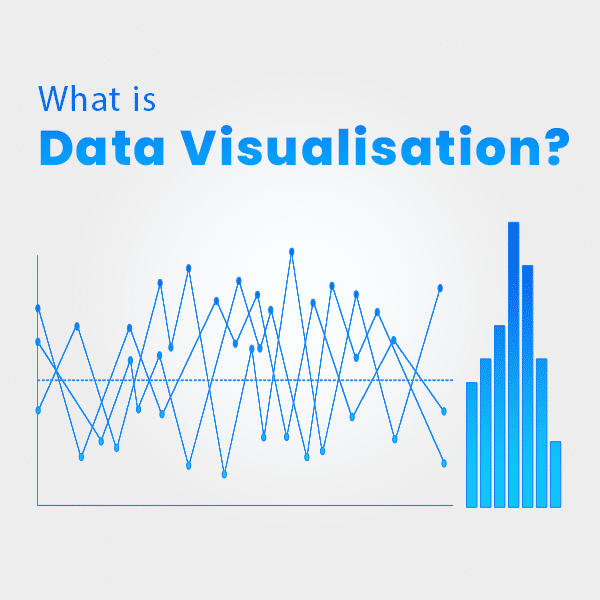
Data visualisation is the process of graphically representing data that can be easily understood by humans. Experts arrange and communicate unstructured data to humans. They represent it in graphs, charts, infographics and other formats with tools and computational techniques.
Businesses across various industry verticals irrespective of size, are dependent on data today. These organisations are constantly churning out data. However, this data is totally raw and is unsuitable for use. Data visualisation enables structuring and graphically representing the data. This helps in drawing crucial insights from it that can help businesses make essential business decisions.
What is the Role of Data Visualisation in Data Science?
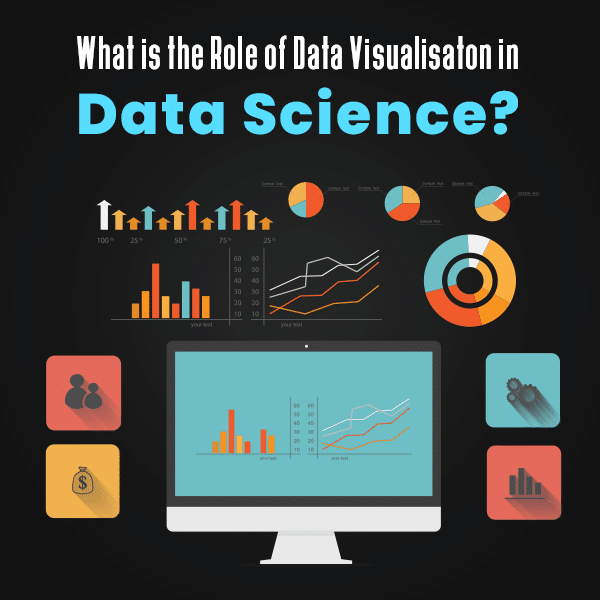
Data visualisation is a major aspect of data science communication. It enables experts to communicate and present useful data to businesses graphically. Data visualisation engineers look for trends and patterns, conduct data cleaning and present structured data.
However, to understand the process of data visualisation, we need to get to the details. Here’s how it helps in various steps associated with any data science project development:
- Data cleaning
Data cleaning is an essential part of data analysis, and visualisation plays a vital role here. Here, data visualisation is crucial for eliminating multicollinearity and detecting outliers. Outliers refer to a data point which differs greatly from the other observations. For detecting outliers, scatterplots are created.
- Data exploration
Data exploration is a prerequisite for building any data model from the unstructured data available. For that, experts use explanatory data analysis. Data Visualisation is a major component of EDA (exploratory data analysis). It enables experts to look into the available data and figure out the variables and relationships between them.
- Providing model information or data about project flow
Data scientists have to inform stakeholders of the status and progress of a project without the complexities. They use the visualisation of data to communicate this information. Multiple accuracy and performance metric are useful in this regard. Also, visualisation offers the stakeholders a peek into the goals and objectives of the data science project.
- Determining trends and communicating results
Data scientists and analysts use visualisation to identify trends and patterns in the data sets. This helps in improved and efficient analysis of the data. Moreover, they can easily communicate the finding results through graphical representations.
Are Data Visualisation and Data Storytelling the Same?
Data visualisation and data storytelling are often discussed in conjunction. However, they are distinctly different from each other. Data storytelling focuses on presenting data to audience through various communication techniques in a neat, simple and concise way. Data visualisation is the presentation of unstructured data in a graphical format.
However, simply presenting the data in graphical representation is not enough. The stakeholders of a project need to understand it in plain language. This is where data storytelling comes in. Without storytelling, even the graphical representation of data visualisation would be only a few lines and dots.
Wrapping up
Data visualisation not only plays a vital role in data science but also finds its application across multiple fields. Its applications include gaming, preparing weather reports, mapping website performances, astronomy and others. Visualisation of data requires multiple tools, skills and programming language knowledge.
However, it is necessary to choose the right tools, software and techniques while keeping affordability in mind.